Five questions for Beth Rudden
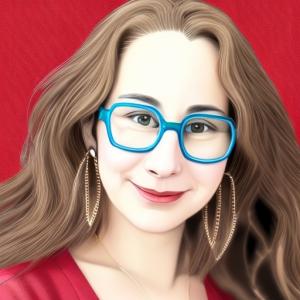
Global IT and cognitive science leader Beth Rudden will be the keynote speaker for the 2023 Colorado Learning and Teaching with Technology (COLTT) conference. Click here to register for the Aug. 3 event at CU Boulder’s Aerospace Engineering Sciences Building on the East Campus.
Rudden has over 20 years of experience in IT and data science. She has driven digital transformation through trusted artificial intelligence (AI) systems for IBM in roles including Distinguished Engineer, Chief Data Officer and Chief Data Scientist. In 2022, she founded Bast.ai, a groundbreaking corporation pioneering the future of conversational AI technology. Its aim is to empower everyone, from students and educators to doctors and marketing professionals, to interact with information in a new way so people can learn and acquire knowledge better and faster.
Rudden earned a master’s in anthropology from the University of Denver and a classics degree from Florida State University. She boasts over 30 patents and numerous publications.
1. How did you become interested in AI, and what do you see as the most compelling points of intersection with higher education – or the ones we should be paying close attention to?
I became interested in AI when I started the Trustworthy Center for Excellence at IBM. We educated our clients and customers on what AI governance was and what AI ethics meant, and we were saying ‘AI’ hundreds of times a day at a time when people weren’t even talking about it yet!
Through this work, we found that education is the number one thing needed. Most people don’t think in terms of probabilities, so we need to make sure that people understand how these models are using large volumes of data that are dubious in nature, with lots of variation, to generate a statistical mean, and that this is dangerous. I knew that education could solve the AI issues of today and it is why I made my way out of IBM to see what I can do to be a part of this solution.
In terms of higher education, I am interested in the position of students and professors on the forefront of the workforce at a time when it is drastically changing. I urge professors to think about how they are using chatGPT and other generative models and how their students are using them. How can professors quickly pivot their thinking to give this next generation what they really need to be successful? Most importantly, how do we collaborate with our students in the use of AI tools to ensure they can help shape a workforce that will be psychologically and physically safe and be contributing members of society?
I think we have a massive opportunity to have our students reflect a newer way of looking at data and measuring what matters and start to measure what we really want to incentivize if we want them to be successful.
2. What are some of the ethical considerations when using AI in higher education?
First, let’s address the elephant in the room: We are not talking about something that has sentience. Therefore, we need to teach our students the rules of engagement for using AI responsibly.
How do we teach students the rules of “ethical engagement” or ethical behaviors when using AI? Three principles should guide decision-making. The first principle is that the intent of AI is to augment humans. This makes sense until we consider all the “net nanny” software that measures how busy or attentive someone is or the software that is using facial recognition to measure and assess someone’s emotion. These are not designed to augment humans; they are designed to control and have power over humans. So how can we build AI that is specifically designed to augment humans? The answer to that is based on the “who” you are trying to augment, and you have to ask, am I enabling and empowering?
A second principle of ethical engagement in AI is that data and insight belong to the creators. The inputs and the responses belong to the user and the community, not corporations. A third principle is that all algorithmic processes should be explainable and transparent. I often get pushback that this is impossible, but it’s not. Transparency and explainability are possible when combining the powers of math with qualitative linguistic and semantic analysis.
3. How do you envision AI transforming the future of higher education, and what opportunities do you see for innovation in this field?
We need to go from the sage on the stage to the guide on the side – from pedagogy to co-creation. This is uncomfortable because it’s a serious responsibility. This goes back to clearly defining the three principles and to ethics – not simply defining the kind of learning goals and naming the learning. AI can give people who become deep experts a “Rosetta stone” – it can become part of the co-creation process to participate and learn with students.
4. How do you see the relationship between AI and traditional forms of teaching and learning evolving over time, and what implications does this have for educators and students?
When the teacher stands in the classroom, they are taking in so many relevant pieces of information – Who’s asking questions? Who isn’t? Who should be? Imagine if you have an augmentation of AI that tells you, “Aisha needs some extra attention today.” It will be positively reinforcing, not negative, and you can apply the principles for building AI that augments. AI makes it possible to have 30 individualized lesson plans to meet unique needs of students. We can also use AI to leverage the expertise of educators globally and the question becomes, “How do we bring in what they are doing into what we are doing?” Diversifying perspectives on certain topics means we can unlearn some fixed ideas to then expand our knowledge and understanding.
5. What are good guiding principles and considerations for critically interrogating what AI generates?
If you fear these tools, I recommend asking ChatGPT questions that you know the answers to. Ask it questions about yourself. Often the answers are wrong, and this makes its limitations and inaccuracy obvious. If we demand lineage and provenance – the ability to triangulate a source and tell its origin story – we increase the value of knowledge. Teach students that anything with that good lineage and provenance and triangulated sources demands proof. Make them remember what we are doing and that we have methods for developing proofs that work every single time. It’s not science if it’s not replicable.
I want to sit down with every educator who is afraid of generative AI and help them to understand it’s their responsibility to add their expertise and be the “stewards” of this large language model and to critically think about where the information comes from.
Also, we need to take into account the environmental cost not being included in the total cost of ownership. Just one model training model for a chatGPT cycle uses all the electricity of New York City for an entire month. We need to teach students how to tap the “power of limits.”